Generative AI and Cybersecurity Operations: The Criticality of Standardization
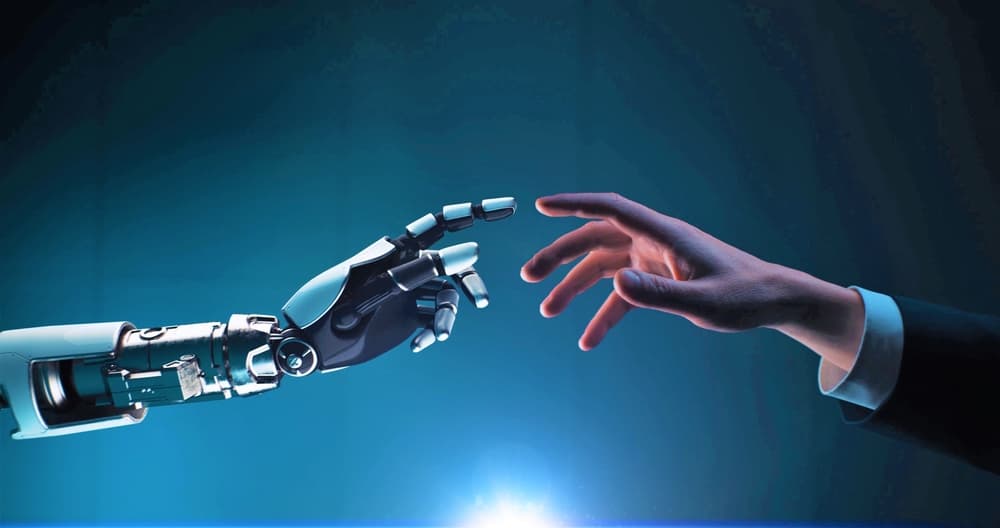
Security Operations • Feb 16, 2024
We use cookies to improve your experience. Do you accept?
Security Operations • Feb 16, 2024
As cyber threats grow more sophisticated and diverse, security operations centers (SOCs) need to leverage a variety of tools and technologies to detect, prevent, and respond to attacks. It is estimated that large enterprises have upwards of 100 disparate security tools. This creates a challenge of interoperability: how can different cybersecurity products and systems work together efficiently and effectively? Today, there is a large amount of excitement around the potential for generative AI to transform cybersecurity operations – but is generative AI alone going to help if we have not first solved our interoperability problems? If I were a Magic 8 Ball, I’d say “Outlook not so good.”
For anyone who may be living under a rock, generative AI is a branch of artificial intelligence that focuses on producing intelligent natural-language responses to questions and tasks, learning from existing data. It has a significant number of cybersecurity applications, from assisting threat hunters with data retrieval for ongoing investigations to providing real-time insights that inform vulnerability management workflows. Generative AI has the potential to improve SecOps in several ways. These include:
Enhancing threat identification. Generative AI can help analysts spot an attack faster, and then better assess its scale and potential impact. For instance, it can help analysts more efficiently filter incident alerts, rejecting false positives. Generative AI can also help detect and hunt threats by producing hypotheses, queries, and indicators of compromise based on the available data and context.
Improving remediation and recovery. Generative AI can help analysts contain, eradicate, and recover from an attack by providing remedy and recovery instructions based on proven tactics from past incidents. Generative AI can also help automate some of the remediation and recovery tasks, such as administrating patches, scripts, or configuration changes to fix the vulnerabilities or restore the systems.
Creating awareness and education. Generative AI can help teams raise awareness and educate stakeholders about cyber risks and best practices. For example, it can generate reports, summaries, and recommendations based on the analysis of the organization’s incidents and security posture. It can also generate training materials, simulations, and scenarios to help users and employees learn how to prevent and respond to cyberattacks.
Generative AI is a powerful tool for cybersecurity operations, but it is no magic bullet. It has some serious drawbacks and challenges, especially when it comes to interacting with other cybersecurity products and systems. One of the main issues is its dependency on APIs and data models.
APIs are the interfaces that enable different products or systems to communicate and exchange data. Data models are the structures that define how information is organized and represented. Both APIs and data models are essential for generative AI to access, understand, and use the data it needs to produce outputs. However, APIs and data models are not normally standardized or consistent across different cybersecurity products and systems. They may vary in terms of design, functionality, format, and protocol. Additionally, APIs are rarely well documented, if said documentation is publicly available at all. This means that generative AI may not be able to learn how to interact with some products or systems. It may produce inaccurate or incompatible outputs if it does not understand or is not able to locate and work with your vendors’ APIs and data models.
Let’s consider this example: If generative AI doesn’t know how to use your SIEM’s API, it may not be able to retrieve relevant data – or worse, if it doesn’t understand the data model, it could unknowingly generate false or irrelevant alerts. Similarly, generative AI may not be able to automate a remediation task using a SOAR system if it does not follow its API – or may create faulty or harmful patches or scripts if it does not adhere to the SOAR data model.
These issues can compromise the efficacy of cybersecurity operations, as well as introduce errors and vulnerabilities. Therefore, generative AI requires careful integration and alignment with existing non-standardized cybersecurity products and systems, which may be costly and complex. Moreover, generative AI models need constant updates and adaptations to keep pace with the rapid evolution of cyber threats and technologies, which becomes more challenging when these updates change data models and APIs.
Cybersecurity interoperability is a major challenge, leading to fragmented, siloed, and inefficient SecOps. The results? Reduced visibility, coverage, and response. A recent Ponemon Institute study confirmed these problems – finding that 53% of organizations use more than 25 different cybersecurity products, and 65% of organizations say that the lack of interoperability among these products is a significant challenge for their security posture. The factors that lead to interoperability issues fall into three main buckets:
Diversity of cybersecurity products and systems. Cybersecurity operations rely on a multitude of tools and technologies, such as security information and event management (SIEM), security orchestration, automation, and response (SOAR), extended detection and response (XDR), and threat intelligence platforms (TIP). These products and systems have different functions, features, architectures, and protocols, making it difficult to integrate and communicate with each other.
Lack of common standards and frameworks. There is no universal agreement on how cybersecurity products and systems should be designed, developed, deployed, and operated. There are some efforts to establish standards and frameworks for cybersecurity, such as the OASIS STIX, TAXII, and CACAO standards, and community efforts like OCSF and the Sigma project – but most of these standards are not widely adopted, and support is rarely mandated during procurement, hindering adoption curves.
Rapid evolution of cyber threats and technologies. Cybersecurity is a dynamic and fast-changing domain, where new threats and technologies emerge and evolve constantly (I’m sure you’ve heard this from just about every cybersecurity vendor – it’s the one constant in the industry). This requires security operations teams to constantly update and adapt their products and systems, which may introduce compatibility issues and vulnerabilities. Furthermore, this creates a gap between the state-of-the-art and the state-of-the-practice, where the latest research and innovation may not be readily available or applicable to real-world scenarios.
While generative AI has the potential to dramatically improve cybersecurity outcomes, it alone is not a panacea. Generative AI and standardization efforts must work together to achieve the optimal cybersecurity outcome: a comprehensive and coordinated defense against cyberattacks, driven by an open ecosystem of tools. AI use cases can benefit greatly from standards, as standards facilitate the integration and alignment of generative AI with the existing cybersecurity products and systems, by providing common terminology, protocols, formats, and interfaces. Without such standards, it is a difficult task to extract the optimal value from generative AI tools.